4.5. Effect size (Pearson’s r)#
The effect size for a correlation is quantified simply by the correlation coefficient Pearson’s \(r\).
Remember an effect size tells us how large the effect of interest is, in comparison to random variation.
In the case of a correlation, the effect of interest is the relationship between \(x\) and \(y\) along the best-fit line \(y=mx+c\), whilst the random variation is the spread of data about this line
Pearson’s \(r\) quantifies exactly that - remember this figure from the section on Correlation in the chapter on Describing Data (you may like to look back at it):
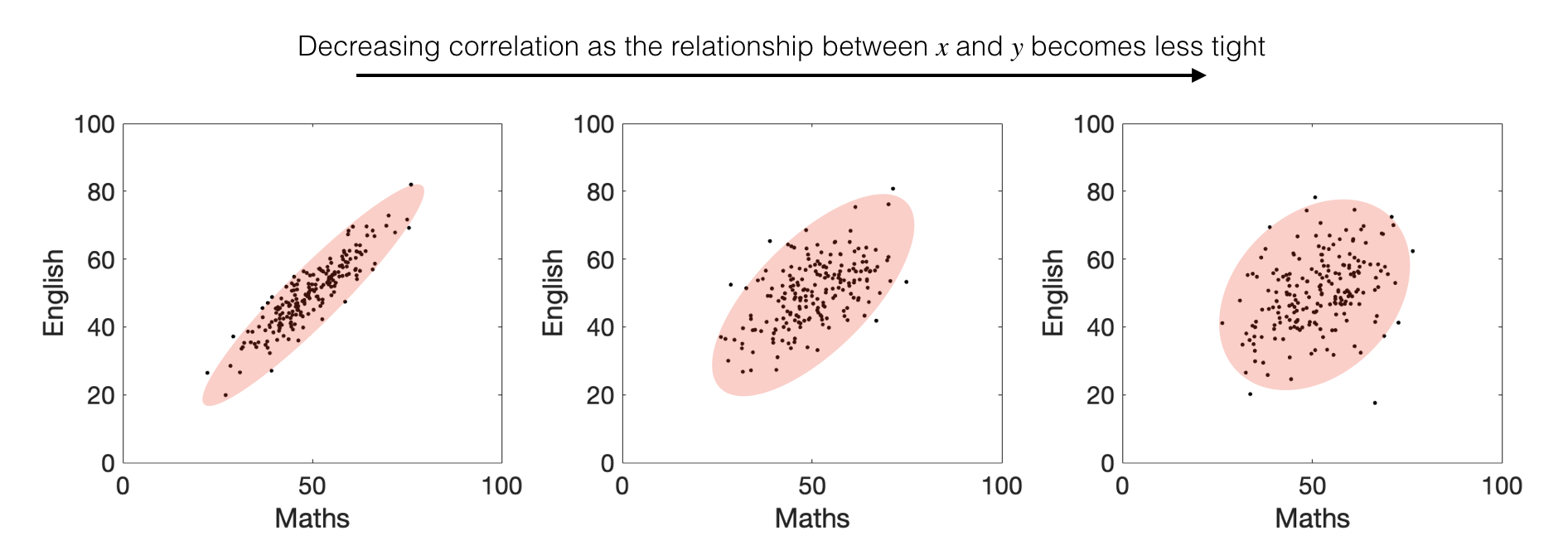
The correlation coefficient \(r\) is always a number between 1 (perfect positive correlation; all points on a line) and -1 (perfect negative correlation)
Correlation gives us a ‘pure’ measure of the strength of the relationship between \(x\) and \(y\) (corrected for the spread within \(x\) and \(y\) independently).
4.5.1. Effect size \(\neq\) statistical significance#
Note that for any given correlation, whether it is believable or statistically significant also depends on the sample size \(n\).
Consider the following examples:
the top row all have sample size \(n=12\)
the bottom row have sample size \(n=200\)
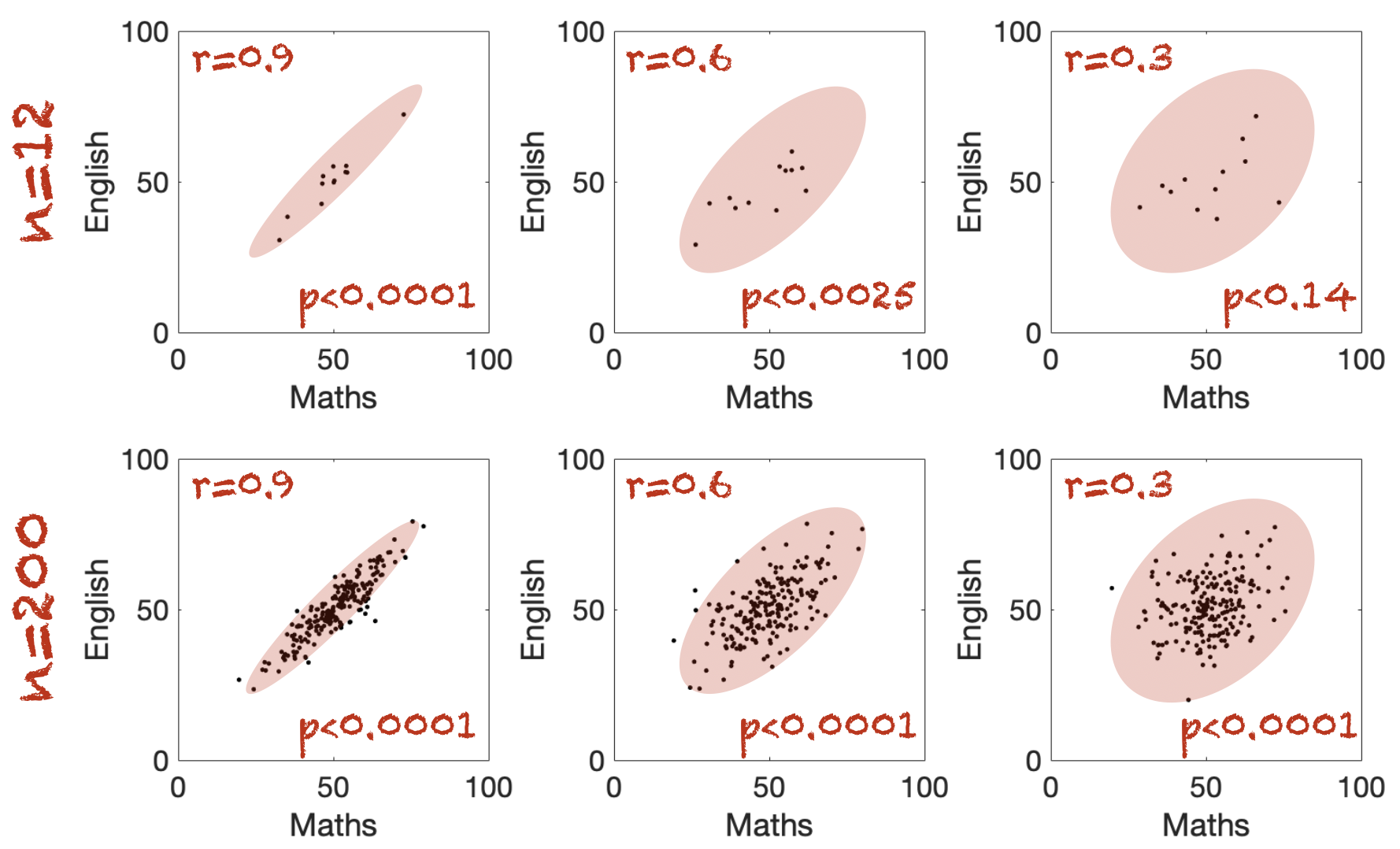
The red oval captures the shape of the dot cloud; an elongated shape is a stronger correlation and greater effect size.
Nonetheless, for a given effect size, the correlation is less convincing for small \(n\) - this is reflected in the statistical significance (\(p\) values).
Statistical significance of \(r\)#
For a given values of Pearson’s \(r\), we can actually test the significance of the correlation using a one-sample \(t\)-test.
We calculate a \(t\)-score using the equation:
and then the \(p\)-value is obtained from the \(t_{n-2}\) distribution.
However you can get straight to the \(p\)-value by using scipy.stats
- note that we are using scipy.stats
as the other correlation functions we met in numpy
and pandas
do not return a \(p\)-value.
Here is the syntax to test the correlation between Maths and English scores, assuming these are columns in a dataframe scores
:
stats.pearsonr(scores.maths, scores.english).pvalue
What counts as a large effect#
Wikipedia tells me that \(r\) values of 0.1, 0.3 and 0.5 are considered small, medium and large respectively
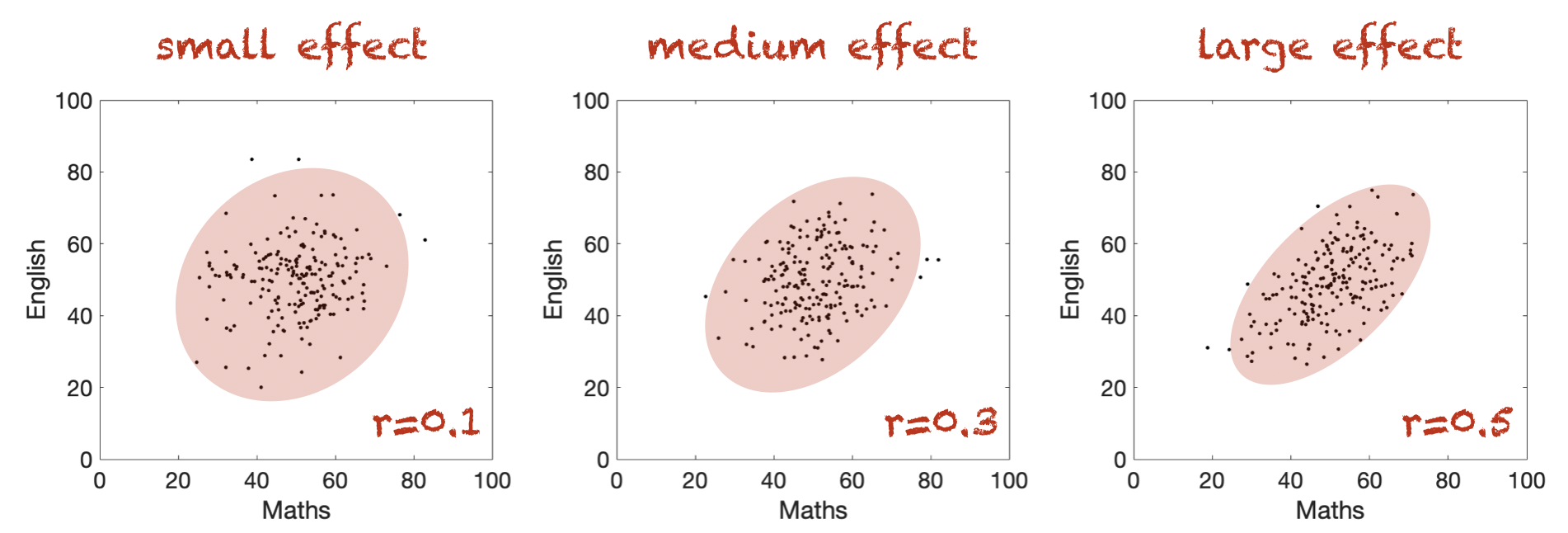
… but bear in mind this is just a rule of thumb.