4.6. Kruskall-Wallis test#
When the sample size is small, or when the data are not normally distributed, it is preferable to use the Kruskal-Wallis test which is the non-parametric equivalent of ANOVA. The equation for the K-W test is:
Where the test statistic, \(H\), has a chi-squared distribution.
In this equation, \(K\) references the number of groups, \(n_i\) indicates the number of observations per group, and \(R_i\) is the sum of the ranks of observations in the group.
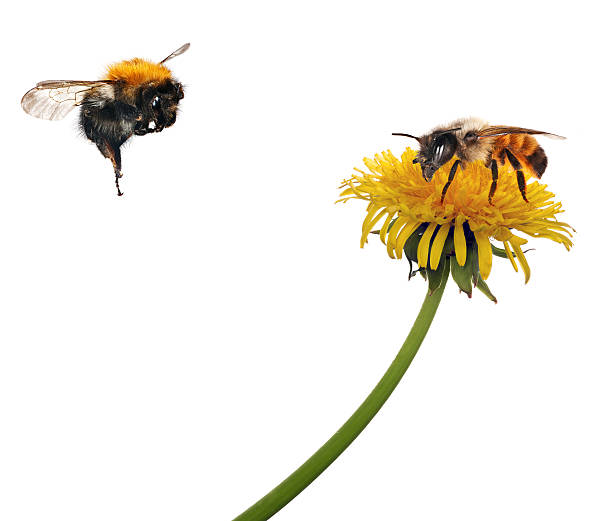
Longhand calculation example: A research paper published in the Journal of Insect Conservation in 2019 examined the attractiveness of different wasteland sites to bees. The table below shows a record of the richness of bee species in 10 wasteland sites, classified by the former land use into extractive industry, suburban/ residential and chemical industry.
Bee Species Richness:
Ob1 |
Ob2 |
Ob3 |
Ob4 |
|
---|---|---|---|---|
Chemical industry |
33 |
27 |
40 |
|
Extractive industry |
95 |
117 |
105 |
71 |
Suburban/residential |
55 |
73 |
67 |
Test whether species richness differs by type of site using the Kruskal-Wallis test.
State your hypotheses.
Click to reveal answer
\(\mathcal{H_0}\): the group rank means are equal, i.e., there is no association between site type and bee species richness.
\(\mathcal{H1}\): at least two of the rank means are unequal, i.e., there is an association between site type and bee species richness.
What are the rank sums for each group?
Click to reveal answer
n |
Rank Sum |
|
---|---|---|
Chemical industry |
3 |
6 |
Extractive industry |
4 |
33 |
Suburban/residential |
3 |
16 |
Practice your ability to work with equations. Plug the values in and calculate H.
Click to reveal answer
With 2 df, the critical value of \(H\) = 5.99 (Look up here again).
As 7.318 > 5.99, we reject the null hypothesis and conclude that there is a difference between groups.