10.7. Permutation testing - more practice#
We will work with the (made up) wellbeing survey data that we used for the exercises on bootstrapping.
The aim of these exercises is to give you some practice running permutation tests and deciding what to permute and what your test statistic is in each case!
Set up Python libraries#
As usual, run the code cell below to import the relevant Python libraries
# Set-up Python libraries - you need to run this but you don't need to change it
import numpy as np
import matplotlib.pyplot as plt
import scipy.stats as stats
import pandas
import seaborn as sns
Import and view the data#
wb = pandas.read_csv('https://raw.githubusercontent.com/jillxoreilly/StatsCourseBook/main/data/WellbeingSample.csv')
wb
ID_code | College | Subject | Score_preVac | Score_postVac | |
---|---|---|---|---|---|
0 | 247610 | Lonsdale | PPE | 60 | 35 |
1 | 448590 | Lonsdale | PPE | 43 | 44 |
2 | 491100 | Lonsdale | engineering | 79 | 69 |
3 | 316150 | Lonsdale | PPE | 55 | 61 |
4 | 251870 | Lonsdale | engineering | 62 | 65 |
... | ... | ... | ... | ... | ... |
296 | 440570 | Beaufort | history | 75 | 70 |
297 | 826030 | Beaufort | maths | 52 | 49 |
298 | 856260 | Beaufort | Biology | 83 | 84 |
299 | 947060 | Beaufort | engineering | 62 | 65 |
300 | 165780 | Beaufort | PPE | 48 | 56 |
301 rows × 5 columns
1. For engineering students, is wellbeing increased after the vacation compared to before?#
We hypothesise that engineering students will feel better after the vacation. Test the hypothesis using a permutation test.
Let’s get just the rows of the dataframe containing engineering students:
wb[wb['Subject']=='engineering']
# OR -
# wb.query('Subject == "engineering"')
# see Sammi's coding support sheet
ID_code | College | Subject | Score_preVac | Score_postVac | |
---|---|---|---|---|---|
2 | 491100 | Lonsdale | engineering | 79 | 69 |
4 | 251870 | Lonsdale | engineering | 62 | 65 |
6 | 841260 | Lonsdale | engineering | 71 | 58 |
7 | 960120 | Lonsdale | engineering | 54 | 54 |
15 | 670880 | Lonsdale | engineering | 70 | 69 |
... | ... | ... | ... | ... | ... |
266 | 842870 | Beaufort | engineering | 52 | 58 |
278 | 414020 | Beaufort | engineering | 76 | 82 |
291 | 384240 | Beaufort | engineering | 78 | 89 |
294 | 457900 | Beaufort | engineering | 72 | 62 |
299 | 947060 | Beaufort | engineering | 62 | 65 |
61 rows × 5 columns
Things you need to decide:#
what is our null hypothesis
what is our alternative hypothesis?
Is it a paired or unpaired test?
therefore which
permutation_type
is needed,samples
,pairings
orindependent
?are we testing for the mean difference (within pairs)
mean(x-y)
, or the difference of meansmean(x)-mean(y)
what actually are
x
andy
in this case?
Is it a one- or two-tailed test?
therefore which
alternative
hypothesis type is needed,two-sided
,greater
orless
?
What $\alpha$ value will you use?
what value must $p$ be smaller than, to reject the null hypothesis?
this is the experimenter’s choice but usually 0.05 is used (sometimes 0.001 or 0.001)
The answers to the things you need to decide!#
$\mathcal{H_0}$: The mean difference in wellbeing post-pre vacation is zero for engineering students
$\mathcal{H_a}$: The mean difference in wellbeing post-pre vacation is greater than zero, ie wellbeing improves over the vacation.
This is a paired test as we are comparing each person’s wellbeing before the vacation to their own wellbeing after the vacation. Therefore we are testing the mean difference mean(x-y)
and need to shuffle the samples
(flip pre and post labels with pairs, but don’t break the pairings)
# define a funtion that gives the mean difference for two series x and y
def mDiff(x,y):
return np.mean(x-y)
It’s a one-tailed test because our hypothesis is directional (we expect wellbeing to increase after the vacation) and if we calculate of difference as post-pre, a significant effect would be a big positive difference, so we need alternative='greater'
We will test at the 5% ($\alpha=0.05$) level
Run the test!#
Here is some code to run the test
post = wb[wb['Subject']=='engineering']['Score_postVac']
pre = wb[wb['Subject']=='engineering']['Score_preVac']
stats.permutation_test((post,pre), mDiff, alternative='greater', permutation_type='samples', n_resamples=10000)
PermutationTestResult(statistic=1.0491803278688525, pvalue=0.22717728227177283, null_distribution=array([-1.21311475, 2.26229508, -0.16393443, ..., 2.55737705,
-0.26229508, 0.39344262]))
Is it significant?#
Reading the test output:
statistic=1.0491803278688525
The mean post-pre wellfare score difference in our ‘real’ sample was 1.049 points (welfare was 1.049 points higher after the vac - this is a small increase as it’s a 100-point scale)
pvalue=0.214
The probability of obtaining such a large increase in welfare due to chance if there was really no effect of the vacation is 21.4%
Conclusions#
We fail to reject the null hypothesis as $p$ is not smaller than $\alpha$
How would you write that up in a journal article?#
We hypothesised that wellbeing increased over the vacation. We compared wellbeing in engineering students (n=61) before and after the vacation using a paired samples permutation test. There was no significant increase in wellbeing after the vacation (mean difference is an increase of 1.049 points, p=0.214, one tailed).
2. Do does wellbeing (before the vacation) differ between history and maths students?#
We hypothesise that students studying different subjects may have different levels of wellbeing but we don’t have an a priori expectation about which subjects will have higher wellbeing.
Test the hypothesis using a permutation test.
Let’s get just the prevacation wellbeing scores for history students an just maths students:
history = wb[wb['Subject']=='history']['Score_preVac']
maths = wb[wb['Subject']=='maths']['Score_preVac']
Things you need to decide:#
what is our null hypothesis
what is our alternative hypothesis?
Is it a paired or unpaired test?
therefore which
permutation_type
is needed,samples
,pairings
orindependent
?are we testing for the mean difference (within pairs)
mean(x-y)
, or the difference of meansmean(x)-mean(y)
what actually are
x
andy
in this case?
Is it a one- or two-tailed test?
therefore which
alternative
hypothesis type is needed,two-sided
,greater
orless
?
What $\alpha$ value will you use?
what value must $p$ be smaller than, to reject the null hypothesis?
this is the experimenter’s choice but usually 0.05 is used (sometimes 0.001 or 0.001)
The answers to the things you need to decide!#
$\mathcal{H_0}$: The difference mean wellbeing pre vacation for history and maths students is zero
$\mathcal{H_a}$: The difference mean wellbeing pre vacation for history and maths students is non-zero, ie one subject has higher wellbbeing
This is an independent samples test as we are comparing each person’s wellbeing before the vacation to their own wellbeing after the vacation. Therefore we are testing the mean difference mean(x)-mean(y)
and need permutation_type = 'independent'
(shuffle which datapoints are labelled ‘history’ or ‘maths’ but keep the number of students labelled as history and maths matching the original sample)
# define a funtion that gives the mean difference for two series x and y
def dMeans(x,y):
return np.mean(x)-np.mean(y)
It’s a two-tailed test because our hypothesis is non-directional (we don’t have particular predictions about which subject we might have higher wellbeing, we are just interested in whether there is a difference) so we need alternative=’two-sided’
We will test at the 5% ($𝛼=0.05$) level
Run the test!#
Here is some code to run the test:
stats.permutation_test((history,maths), dMeans, alternative='two-sided', permutation_type='independent', n_resamples=10000)
PermutationTestResult(statistic=3.486388384755003, pvalue=0.17638236176382363, null_distribution=array([ 4.61887477, 2.00544465, 0.87295826, ..., 0.52450091,
-0.43375681, -2.43738657]))
Is it significant?#
Reading the test output:
statistic=3.486388384755003
The difference in mean welfare score in our ‘real’ sample was 3.49 points (welfare was 3.49 points higher for history students)
pvalue=0.16718328167183283
The probability of obtaining such a large difference in welfare due to chance if there was really no effect of the subject is 16.7%
Conclusions#
We fail to reject the null hypothesis as $p$ is not smaller than $\alpha$
How would you write that up in a journal article?#
We hypothesised that wellbeing might differ between students in different subjects. We compared wellbeing in maths students (n=29) and history students (n=19) before the vacation using an independent samples samples permutation test. There was no significant difference in wellbeing between subjects (difference of means = 3.48 points, p=0.167, two tailed).
Out of interest, why was this large difference not significant?#
Probably because tha variation in welfare within each group was relatively large, and the sample size was relatively small:
sns.histplot(history, color='b', alpha=0.5)
sns.histplot(maths, color='r', alpha=0.5)
plt.show()
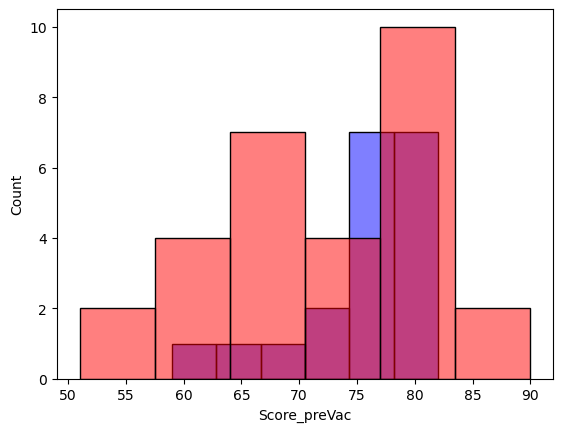